News
Jan 16 '25
FoodAtlas: An AI-driven Knowledge Base of Food, Chemicals, and Health Effects
#AIFS
#Education
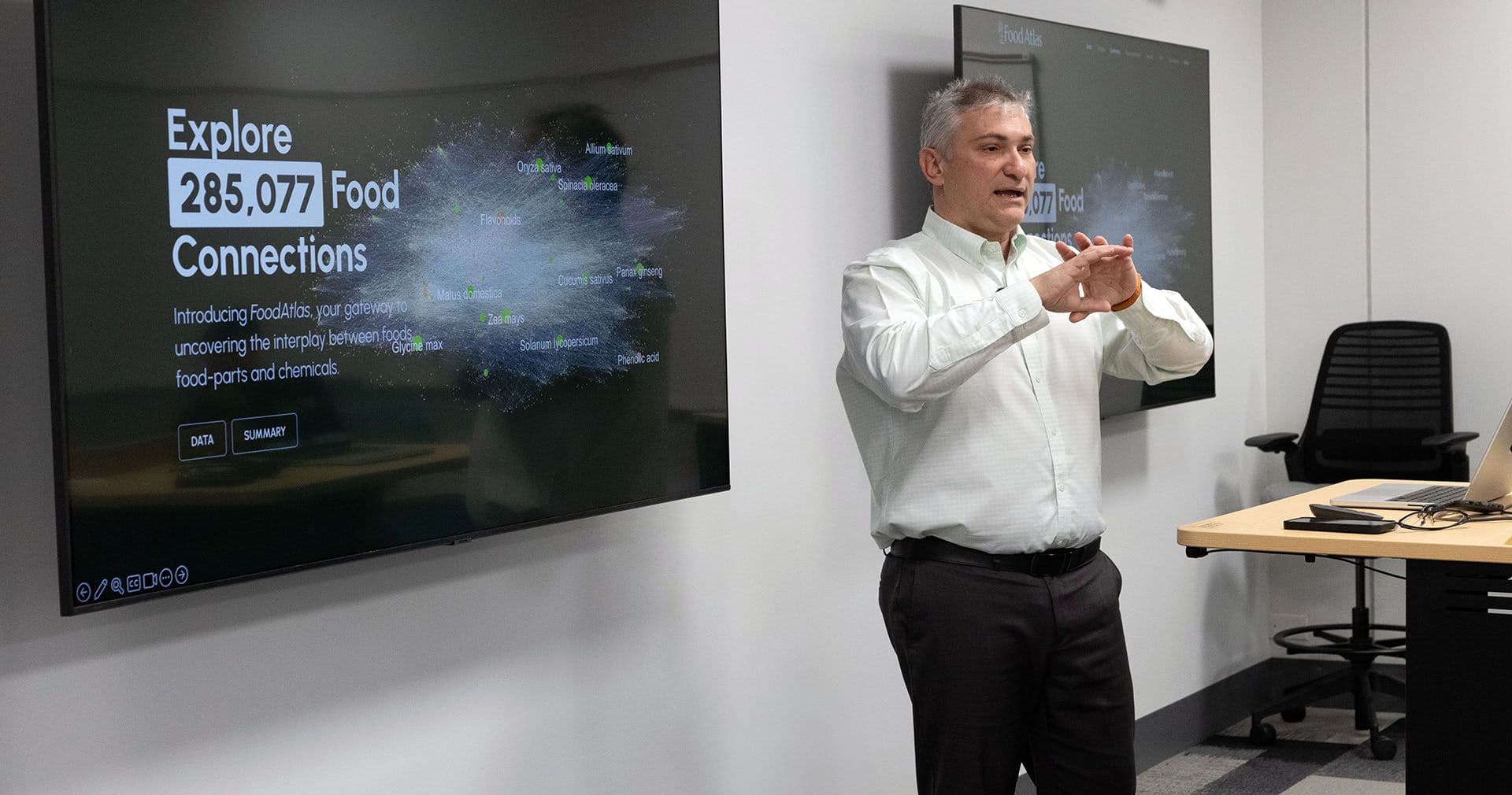
Ilias Tagkopoulos opens with a challenge for the audience. “Think about what you ate this morning, what you ate yesterday. Are you happy? Are you happy with your choices? Do you think you have the information you need to make healthy choices?”
It turns out we don’t really know enough about what’s in our food and what those foods are doing to our bodies and minds.
A few years ago, it was reported that we only knew 2% of what is in our foods. And we’re talking about the raw ingredients – plants and meats before any processing. Over the past few years, we’ve been learning more. Now, we estimate the percentage of known food composition to be between 5 and 10%. When we ask what these chemicals, the components of foods, do to our body, even less is known.
In order to develop healthier foods that people will choose to incorporate into their diets, we need to understand what's in our food, what it does to our body, and the characteristics of flavor and texture.
The current go-to resource to learn what’s in our food is FooDB. While this is an amazing resource and the best available to date, less than 1% of entries link back to the knowledge source. It is not connected to evidence verifying the information is scientifically correct.
Another notable source is USDA’s FoodData Central Foundation Foods. They have very good information, but out of tens of thousands of foods, they focus on only 236.
Thousands of evidence-supported food-chemical associations are found in these databases, but it is estimated that more than a million are reported in public scientific literature.
FoodAtlas was created to address two main issues: 1) to find all known food composition knowledge and organize it in one place, and 2) to make sure the information is scientifically backed with links to the evidence or paper where the knowledge was reported.
How do we do this? Dr. Tagkopoulos provides an introduction to neural networks used in AI and how they model the neurons in our brains. From this, we can create the representation of any possible function. As humans, we’re conditioned to associate things. The neural network learns to make associations, too, and this is where its amazing power comes from.
In the past, reviewing all of the published papers on food composition took 100s or 1000s of hours, time manually spent by undergraduate students to establish reliable data sets. This data was used to train AI models to review literature on its own, with many iterations to develop the level of accuracy desired.
With FoodAtlas version 1, Dr. Tagkopoulos’ team extracted 230,000 food-chemical composition relationships from 155,000 published papers. For example, this food contains this chemical (micronutrient, protein, etc.). It turns out that 46% of these relationships had never been reported in any database!
The world is investing money, doing research, and publishing papers. But much of that knowledge is hidden – lost in a scientific journal that sits on the internet.
We need AI-powered tools to gather all of this information and organize it in a way that can be useful with evidence synthesis. In this case, we can use the knowledge for new products, for new interventions, and for new treatments.
The knowledge of what’s in our food has real-world implications for our health. With scientifically-verifiable data, researchers can use the information to assist in developing new treatments.
AI helps science progress in two ways:
- Increase productivity
- Accelerate innovation
With FoodAtlas, the team is using AI to increase productivity. They are able to review hundreds of thousands of articles in a short amount of time.
Dr. Tagkopoulos goes on to describe specific examples where AI has accelerated innovation – leading to new treatment modalities and better health outcomes. One project identified a biomarker to test if the prescribed diet for Irritable Bowel Syndrome will work for a patient or not. Another looked at E. coli and used AI modeling to help identify 15 antibiotic-resistant genes.
The team also looked at the bioactivity of food compared to that of the chemical components we know about. If an apple reduces inflammation by 30%, do the beneficial chemicals we know it contains also reduce inflammation by 30%? Not really.
This means we still don’t know exactly what it is in an apple that is producing the positive effect. We need to explore more and learn more.
AIFS is working in collaboration with the Periodic Table of Food Initiative, or PTFI, to help expand our knowledge of culturally diverse foods. PTFI is conducting a thorough lab analysis of 500 foods to see exactly what is in them, and this new information will be included in the FoodAtlas database.
It’s one thing to know what is in our foods, but we don’t eat individual foods – we eat meals made up of a combination of foods. People don’t like to change their habits, but could we identify one thing in our diet that could be substituted for a healthier option — and still taste good?
In another project, Dr. Tagkopoulos’ team looked at meals using one million recipes, including information from USDA’s What We Eat in America survey. They made one ingredient substitution, then looked at how much better the bioactivity would be, how much more protective the meals would be of health – and the results were amazing. In this example, they did not take into account flavor, but it is a proof of concept that research in this direction could have a significant impact on human health.
What does the future look like?
We talked about food, but it’s not just the food itself. We need to know how to process it better, how to cultivate the crops, and how to modify the seeds. We need to continue our work.
AI tools combined with evidence synthesis will help – for us and for our planet.