News
Oct 02 '24
What’s In Our Food? An AI Approach to Gather What We Know
#AIFS
#Research
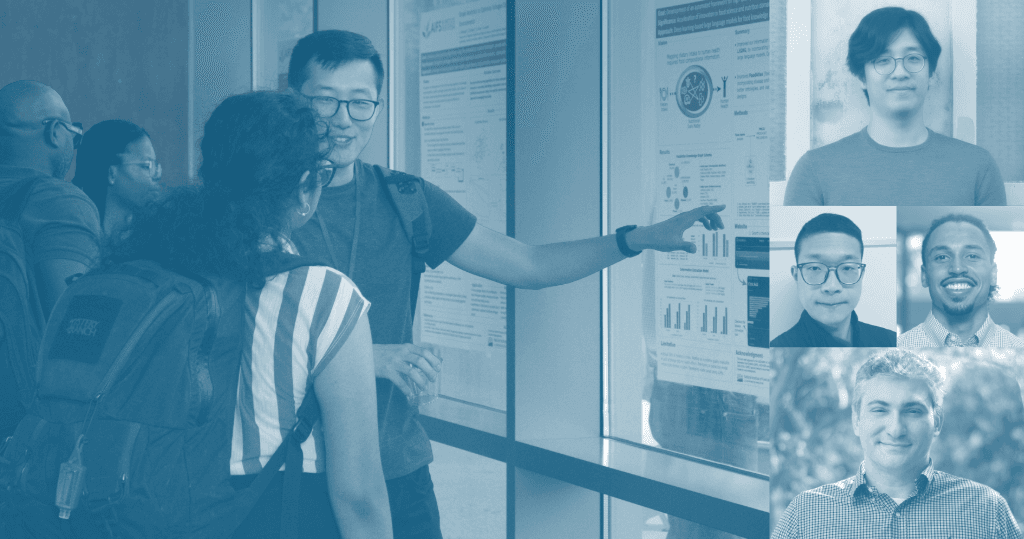
Understanding food composition, including macronutrients and micronutrients, is essential for improving our diet and health.
According to the Centers for Disease Control and Prevention, annual healthcare expenditure for diet-related diseases exceeds $170 billion. Despite the need, only a fraction of chemicals in food have been identified, and about 90% of them remain unquantified.
Much food knowledge is scattered across the scientific literature in unstructured text, but the sheer volume makes it impractical to extract manually. For example, PubMed Central (PMC), a biomedical and life sciences bibliographic database, contains around one million scientific articles on food composition. If human experts were to manually curate food-chemical information from these articles, it would take centuries!
FoodAtlas: Automated Knowledge Extraction of Food and Chemicals From Literature, a recent AIFS-funded publication, describes the research team’s initial approach to tackling this challenge.
The team, led by Jason Youn and Fangzhou Li, developed an AI framework to extract high-quality food knowledge from unstructured text data. Specifically, they fine-tuned a BioBERT language model to do textual entailment, which learned to extract a “{food} contains {chemical}” association given a sentence from an article. They standardized the extracted information and constructed FoodAtlas, a knowledge graph representing the connections between foods and chemicals.
FoodAtlas has two immediate applications. First, it serves as a knowledge base for food, providing sentence-level evidence of a given food-chemical relationship. Second, it can accelerate scientific discoveries.
In one analysis, the team showed that a machine learning model trained on FoodAtlas could discover previously unknown relationships between foods and chemicals. This is particularly interesting for drug development, which is known for its expensive procedures, where FoodAtlas can help scientists speed up finding novel food sources for natural product therapeutics.
What’s next?
FoodAtlas has been actively under development, and many improvements have been made beyond the recent publication of the first version. Specifically, FoodAtlas has adopted the more advanced large language models (i.e. OpenAI GPT-4), extracted chemical concentration values, extended connections to diseases, and incorporated ontologies for easier searches.
Visit foodatlas.ai to explore the most recent results or visit ScienceDirect to review the publication.