News
Feb 11 '25
New Public Data Hub Paves the Way for Safer Food Using AI
#AIFS
#Research
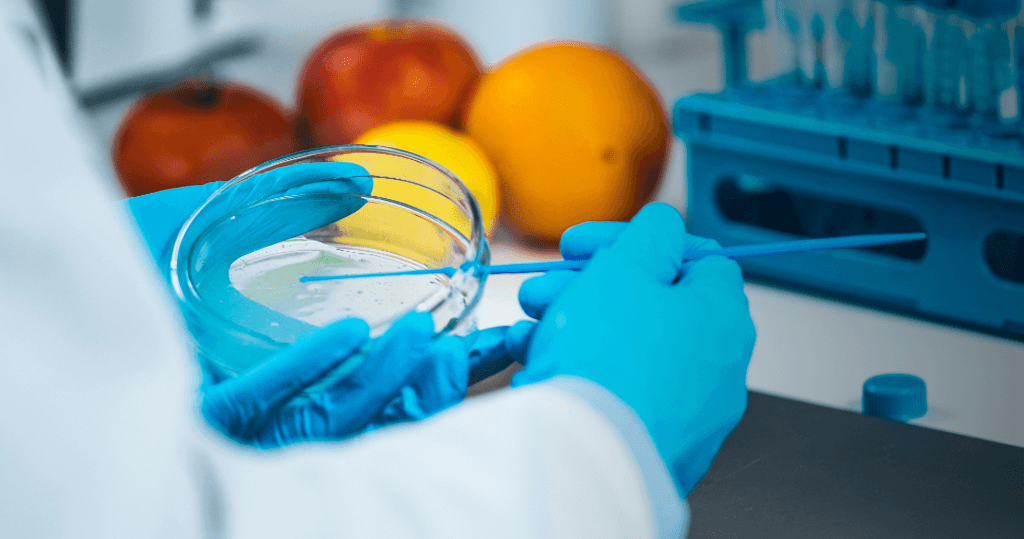

Imagine being able to predict food safety problems before they happen, making our food supply safer and reducing the risk of foodborne illnesses. A new research project is taking a big step toward that goal by creating a public data hub that anyone—researchers, companies, or students — can use to build and test artificial intelligence (AI) and machine learning (ML) models for food safety.
The Problem: Lack of Data to Predict Food Safety Hazards
Food safety is a major public health concern and also a big business risk for food companies. Despite this, the food industry has been slow to adopt new technologies like AI and ML. One key reason is that there aren’t enough well-organized, publicly available datasets to help build reliable prediction models due to challenges with collecting microbial data. Other fields, like agriculture and health, have benefited from centralized databases that provide high-quality data for researchers. Without similar resources in food safety, computer scientists and data experts have struggled to create tools that can forecast outbreaks or contamination events.
Creating a Public Database to Improve Food Safety Predictions
To address this gap, researchers have created the Cornell Food Safety ML Repository — a new public online library of datasets focused on food safety. They took three previously published collections of data and improved them by filling in data gaps, standardizing the format, and carefully annotating the entries with relevant details. Here’s a quick look at the three datasets:
- Soil Samples and Listeria: This dataset includes soil samples collected from different locations across the U.S. It shows whether the bacteria Listeria is present or not and comes with additional details of the sampling location like soil properties, climate data, and surrounding land use.
- Chicken Carcasses and Pathogens: This set comes from tests on young chicken carcasses in processing facilities. It tracks the presence or absence of harmful bacteria like Salmonella and Campylobacter and adds extra information about weather conditions and the timing of the tests.
- Watershed Contamination: Focused on water quality in New York watersheds, this dataset notes fecal contamination and E. coli levels. It also includes data on water characteristics, land use, and weather conditions.
By refining these datasets, the team made them more useful for developing AI/ML models to predict where and when food safety issues might occur.
Testing the Data: Building Early Models
To show how these datasets can be used, the researchers also created some basic machine learning models. They ran tests to see how well these models could predict food safety issues based on the data provided. For example:
- Listeria in Soil: One model, using a method called a Gradient Boosting Machine, performed very well with a high accuracy score. This suggests that it could eventually help predict where Listeria is more likely to be found.
- Chicken Carcass Testing: The models for predicting Salmonella and Campylobacter in chicken carcasses did not perform as strongly, indicating that more detailed data may be needed for these cases.
- Fecal Contamination in Water: Another model using a Gaussian Naïve Bayes approach showed promising results in predicting fecal contamination in water.
Even though some models need further improvement, these early tests demonstrate that having a central, well-organized data repository can spark the development of useful predictive tools for food safety.
Why It Matters
This work can help keep us all healthy – imagine fewer cases of “food poisoning,” Salmonella, or Listeria. Safer food means fewer foodborne illnesses and less economic loss due to outbreaks. Companies can find solutions in this platform to address their existing food safety issues, and subsequently generate more and better data that will further improve the models. With better data, they can monitor their food production more effectively, and public health officials can respond more quickly to potential risks.
By creating a public repository, the research encourages further sharing and standardization of food safety data. This can lead to more advanced AI tools in the future, which could be used to prevent contamination and protect public health.
Another important benefit is the potential to test new methods for keeping data private. Food companies often worry that sharing their data could hurt their reputation or business. With techniques like differential privacy and federated learning, it might be possible to share useful data without exposing sensitive information. This balance could help more companies contribute to the repository, leading to even better models and safer food for all.
The Cornell Food Safety ML Repository is a promising first step toward using AI to make our food safer. By providing well-documented standardized data, it opens the door for researchers and industry professionals to build and improve predictive models. This effort not only has the potential to reduce foodborne illnesses but also to foster a culture of data sharing and innovation in food safety.
This research was funded by the AI Institute for Next Generation Food Systems with the support of USDA-NIFA award #2020-67021-32855. The research team includes Chenhao Qian, Huan Yang, Jayadev Acharya, Jingqiu Liao, Renata Ivanek, Martin Wiedmann.
Read the full Journal of Food Protection article on ScienceDirect.